How To Prototype with AI? [Step-by-Step Guide]
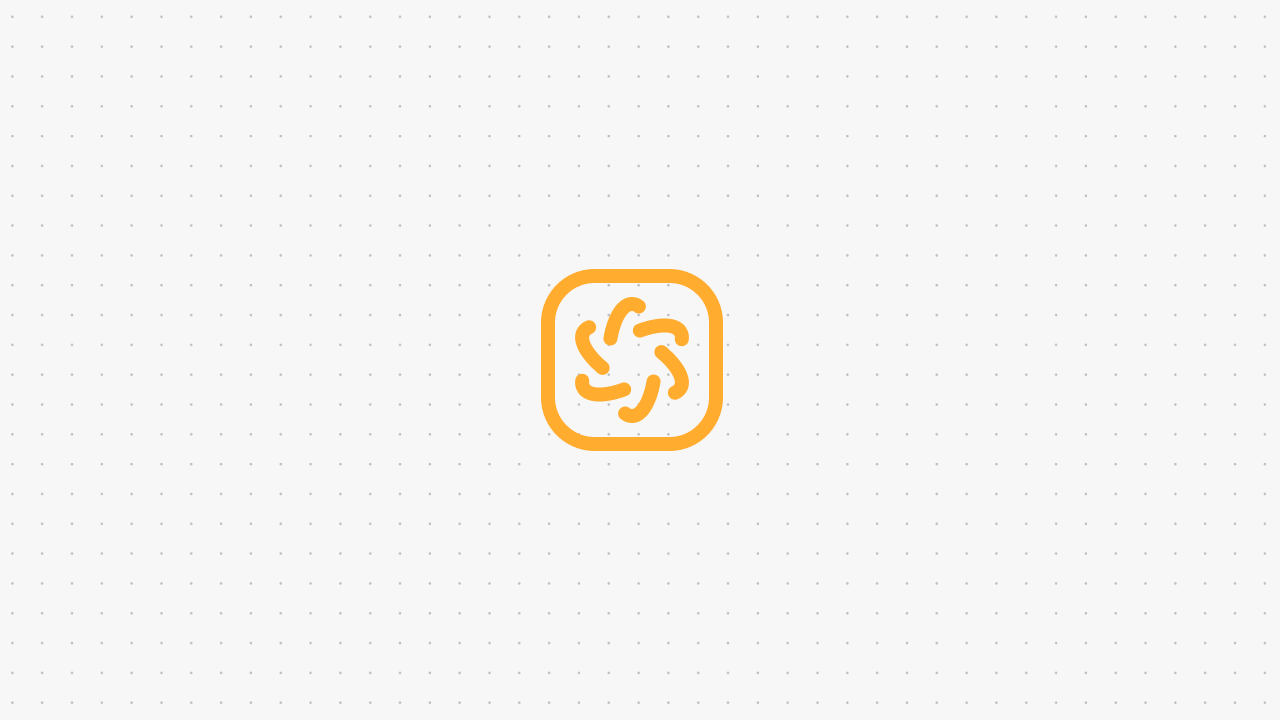
In early 2024, Nvidia unveiled Project GR00T, an initiative aimed at streamlining the development of humanoid robots through advanced AI models. This announcement highlights a broader trend in AI prototyping, where companies increasingly rely on prototypes to accelerate innovation across industries.
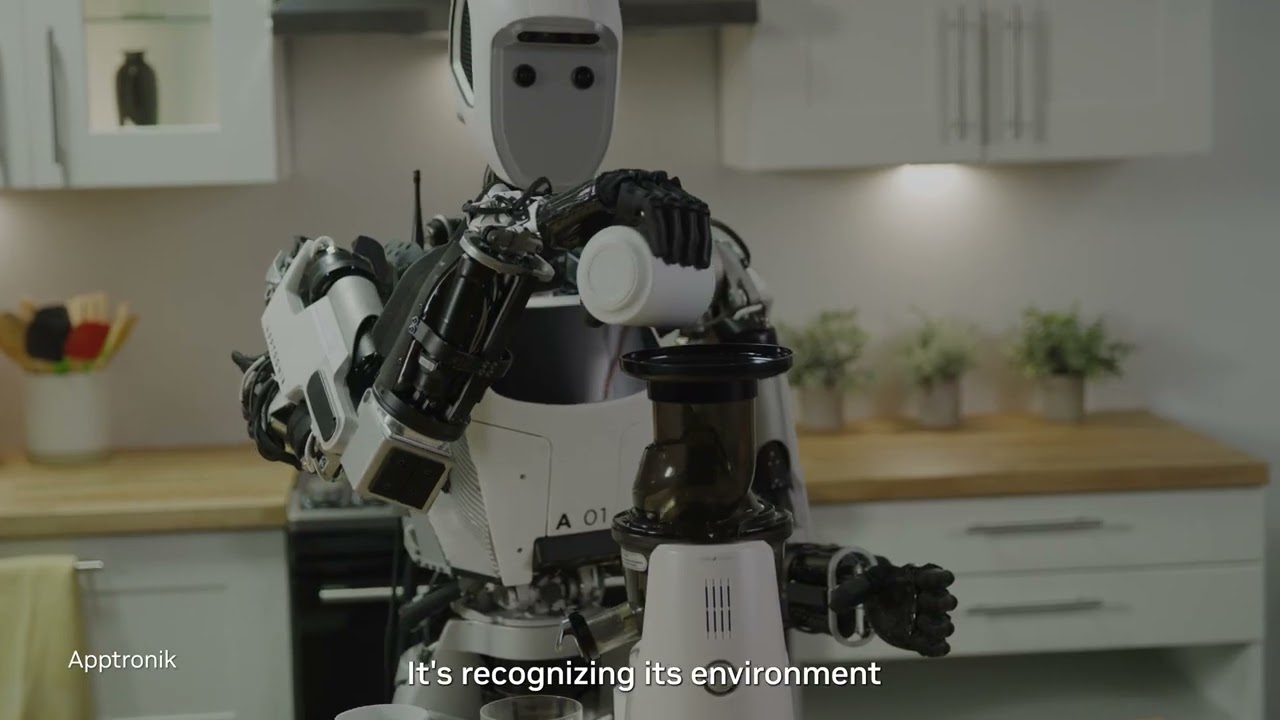
With AI spending projected to grow as 67% of businesses plan to increase investments over the next three years, understanding AI prototyping is more relevant than ever. Let’s dive in.
What Is an AI Prototype, and How Does It Differ From Traditional Prototypes?
An AI prototype is a preliminary model designed to test AI-based solutions. Unlike traditional prototypes, which are static or rule-based, AI prototypes evolve through machine learning, adapting to new data and environments. They can simulate complex decision-making processes, offering deeper insights earlier in the development cycle.
Key differences between AI and traditional prototypes include:
- Dynamic Learning: Traditional prototypes operate on predefined logic; AI prototypes improve over time.
- Data-Driven: AI prototypes rely heavily on data, while traditional ones depend on fixed parameters.
- Scalability: AI prototypes can handle diverse and growing datasets, making them more versatile.
AI Prototyping Process: From Concept to Product
The journey from a visionary AI concept to a market-ready product involves several crucial stages:
- Problem Definition: Clearly articulate the issue the AI is designed to solve
- Data Collection and Preparation: Gather high-quality, relevant data and preprocess it for model training
- Model Development: Select appropriate algorithms and train the model
- Prototype Building: Implement the model into a functional system
- Testing and Iteration: Continuously refine the prototype based on feedback and performance metrics
- Scaling and Deployment: Prepare the prototype for full-scale implementation
In industries like healthcare and finance, AI prototypes are revolutionizing risk assessment, fraud detection, and personalized medicine.
Why Should Businesses Consider AI Prototyping?
In today's fast-paced business environment, AI prototyping offers numerous advantages:
- Reduced development costs and risks
- Faster time-to-market for AI solutions
- Improved decision-making based on data-driven insights
- Enhanced product innovation and differentiation
As businesses increasingly recognize the potential of AI, the need for efficient prototyping
becomes more apparent. This is particularly true in the realm of customer service, where AI agents are revolutionizing the way companies interact with their customers. Voiceflow offers a powerful platform for businesses to prototype and implement human-like AI agents for customer support, enabling companies to stay ahead of the curve in this rapidly evolving landscape. Don't wait – sign up for Voiceflow today and start transforming your customer service experience!
What Industries Benefit Most From AI Prototyping?
In today's fast-paced business environment, AI prototyping offers numerous advantages:
What Tools Are Best for AI Prototyping?
What Industries Benefit Most From AI Prototyping?
Choosing the right tools is crucial for successful AI prototyping. Some popular options include:
- TensorFlow: An open-source platform for machine learning
- PyTorch: A flexible deep learning framework
- Scikit-learn: A machine learning library for Python
- IBM Watson: A suite of AI services and applications
- Google Cloud AI Platform: A comprehensive set of AI and machine learning tools
However, when it comes to prototyping AI agents for customer service, Voiceflow stands out as the premier choice. Its intuitive interface and powerful features make it the ideal platform for businesses of all sizes to create and deploy human-like AI agents. By leveraging Voiceflow, companies can rapidly prototype and implement AI-powered customer support solutions, staying ahead of the competition in today's AI-driven landscape.
How Do Costs for AI Prototypes Compare to Full-Scale AI Solutions?
AI prototypes are significantly cheaper than full-scale solutions, as they focus on proving feasibility rather than full functionality. The cost includes:
- Hardware and Software: Limited infrastructure required during prototyping.
- Development Time: Prototypes are developed faster, reducing labor costs.
- Scalability: Full-scale solutions incur additional costs for scaling and maintenance.
How Do You Measure the Success of an AI Prototype?
Measuring the success of an AI prototype is crucial for determining its viability and potential impact. Key metrics to consider include:
- Accuracy: How well does the prototype perform its intended task?
- Efficiency: Does the prototype complete tasks faster or more efficiently than existing solutions?
- Scalability: Can the prototype handle increased data volumes or more complex tasks?
- User feedback: How do potential users respond to the prototype?
- Return on Investment (ROI): Does it offer measurable financial or operational benefits?
The Urgency of AI Agents for Businesses
AI prototypes often lead to the development of intelligent AI agents. According to Stanford’s Human-Centered AI Institute, 2024 will see a rise in AI agents capable of handling complex tasks autonomously. These agents are transforming customer service by resolving queries in real time, enhancing customer satisfaction, and cutting operational costs.
Voiceflow offers a powerful platform for businesses to prototype and implement human-like AI agents for customer support, enabling companies to stay ahead of the curve in this rapidly evolving landscape. Don’t wait—empower your customer support with AI today. Sign up for Voiceflow and stay ahead of the competition.
Start building AI Agents
Want to explore how Voiceflow can be a valuable resource for you? Let's talk.
