Where we’ve been and where we’re going—in less than 300 words
Some of us are still fighting gray hairs from last year’s stress. AI hype took off in 2023 when ChatGPT became the fastest consumer app to reach 100 million users in just two months. It was a sensation. Executives immediately wanted to know how to use it and whether they should invest in it. Suddenly, teams were under pressure to develop proof of concepts (POCs), compare models, and develop prompt engineering and fine-tuning techniques.
This was also the time for many businesses when stalling in their AI maturity became commonplace. Agents already had a pretty bad rap, and you didn’t want yours to be counted among them, so the risks of innovating were too high for many organizations.
By 2024, we had established our baseline of what AI had the potential to do. But many teams, from big enterprises to small organizations, had questions about ROI, integrations with legacy systems, and how AI would affect existing workflows. How could AI lift the entire organization, rather than just a few teams?
Today, treating your AI agent like you would a core product offering is the best way to ensure you’re creating real solutions to organizational goals—not just slapping AI onto an existing problem.
[4 benefits of treating AI agents as core products, instead of one-off solutions
- Building a dedicated, collaborative team
- Plugging your agent into useful business data
- Starting and scaling across use cases
- Tailoring your agent to your business needs with guardrails]
Mapping your AI product journey, from business case to AI roadmap
Getting your AI agent to production is not an easy step. Denys often runs into customers who have a cool proof of concept and even the backing of their leadership, but they’ve been slow to scale into production or release new use cases for their agents. That’s because, in an enterprise context, the list of contingencies is long—risk, security, budgets, and even the mood of your CEO.
According to Denys, your AI agent needs a solid business case to move forward, “Propose bringing in stakeholders as early as possible to build, iterate, and deploy together. The strongest way to push past the obstacles to production is to create an unbeatable business case where the leadership teams cannot say no—and where security, risk, finance, or any other team’s critical questions are addressed as you build the agent together.”
For example, your business case should answer the question of whether your AI agent will augment existing support or replace it altogether. Roam’s AI agent automates customer support, answering FAQs about their novel approach to the rental car industry. On the other hand, Tico augments existing human support staff by pulling up relevant information and providing answers to customer questions. Both are valid approaches to AI agents, but vastly different, and knowing how your AI agent solves organizational issues is just as important as knowing which issues it solves.
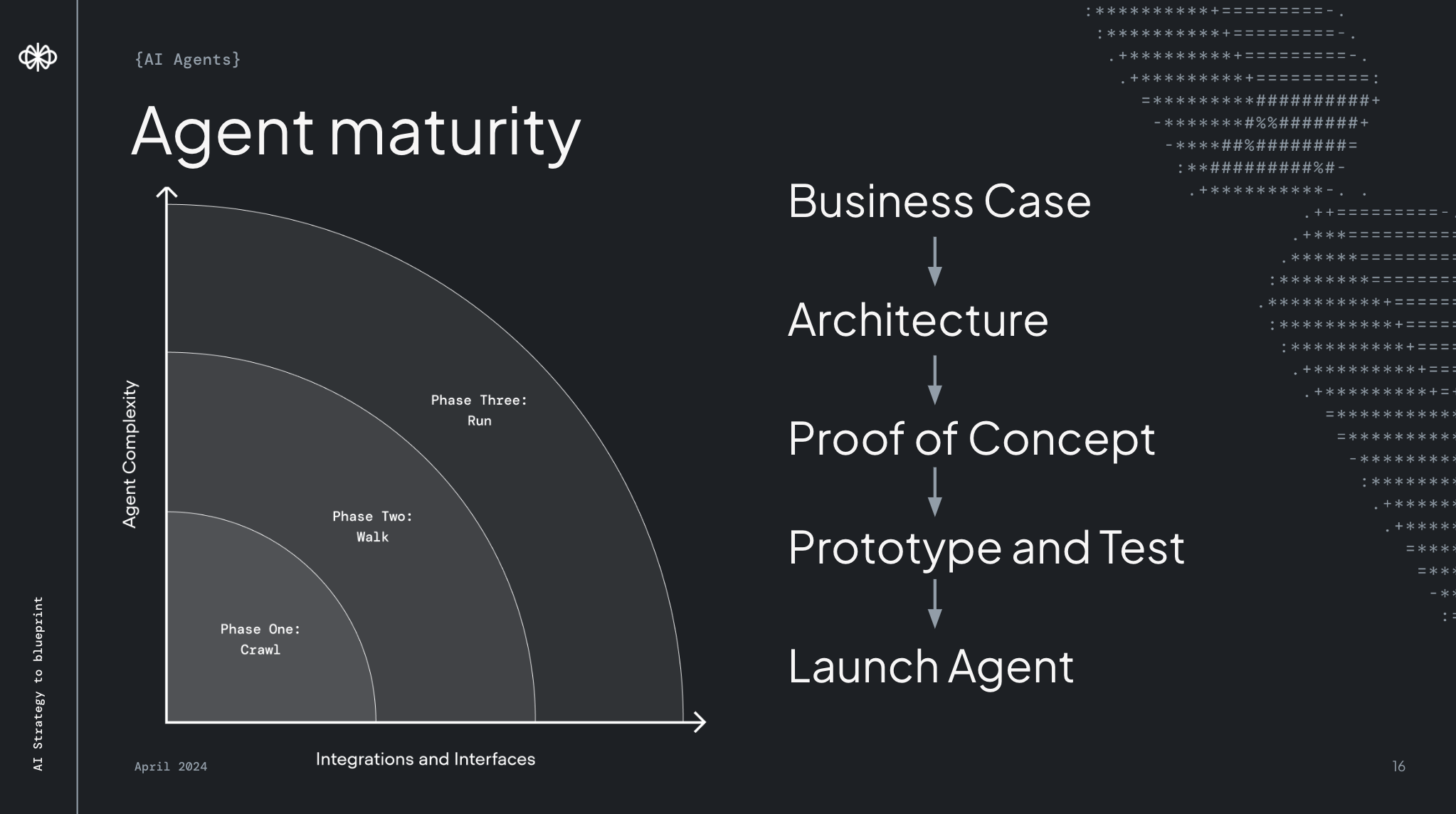
It should be clear by now that getting your agent to production is not a singular step. It’s more like five steps, comprised of building your:
- Business case
- Agent architecture
- Proof of concept
- Prototype for testing
- Launch-ready AI agent
“Ever seen a baby try to get up and run? They fall flat,” Denys explains, “Your agent is that baby, and your steps—from building your case to proof of concept and testing—are all in service of crawling in your AI maturity.” Once you’re comfortably crawling—an AI agent is live, meets a use case, and delivers value—you can begin the toddling steps towards walking, then eventually running. The crawl stage of AI maturity takes some effort, but it's required to deliver on that grand vision of walking or running with complex AI agents.
Think you’re ready to run with your idea? Denys would advise you to slow down and work through it step by step, rather than skipping ahead. “You’ll end up with a wonderful POC that doesn’t leave testing. Build the muscles of a solid production run and start with the foundations first. Every organization can run eventually, but few start there.”
Understand, decide, respond—your AI agent roadmap
Now, let’s say you already have an agent in production (or are close to launching one) and you’re ready to think beyond your initial use cases. We often get questions about how to determine when it’s time to upgrade your AI agent.
One helpful way of mapping the course of your agent is to ask how effectively it currently understands, decides, or responds (UDR), and how you wish to expand on those abilities. Depending on your answers, you can prioritize future use cases that align with those actions and focus on optimizations that solve current blind spots.
Here are some UDR questions to help you create your AI agent roadmap:
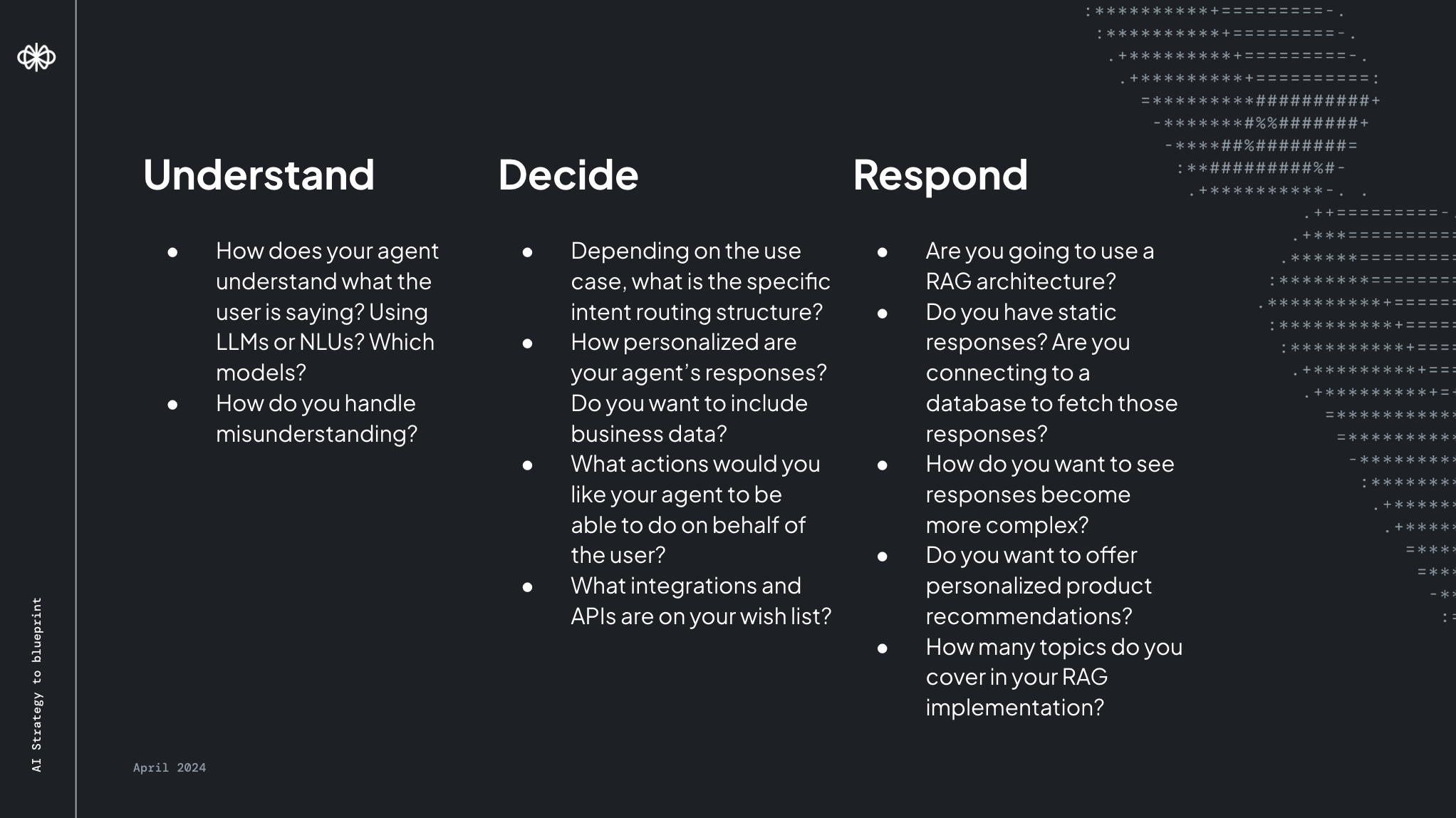
By mapping your agent’s abilities across those three categories, you’ll also better understand who in the organization needs to be involved with production and iteration. Components like access to customer data or your agent’s ability to complete tasks using API integrations will require the expertise and buy-in of multiple team members. Their support will help keep your AI agent out of collaborative gridlock and reduce your time to production.
Your applied AI team, from key roles to upskilling
Any single person can spin up a decent proof of concept. It takes a collaborative team to create a useful AI agent that applies integrations, security, and conversation design. It’s why Denys doesn’t suggest building your AI agent without a team. For your organization to be successful you’ll need many different skills, aligned across functions. In order to do that, you need five roles represented on your AI team:
- Product: They prioritize projects and have a comprehensive understanding of customers and what they need.
- UX/UI: They improve and rebuild workflows. They focus on user research and understanding challenges with current workflows.
- Front end: They integrate the AI agent with your organization’s overall customer experience.
- Back end: They build your AI APIs and integrate your agent with your internal systems.
- Machine learning engineer: They benchmark your current AI maturity, evaluate successes/setbacks, and choose the right AI models.
But what happens when you lack the skills and expertise internally to fulfill these roles? Fear not, here’s a list of five ways to help upskill your current AI team.
How to upskill your AI team
- Courses: These help your team build a foundation with hands-on exercises. Courses equip the whole team with a baseline of AI experience, not just the ML engineer.
- Conferences: Depending on the skill level of your team, conferences can offer new knowledge. These often go into deeper topics, so your team would benefit from having a foundation of knowledge so they know what to ask during discussions with peers. They're also great for networking.
- Hackathons: After establishing a baseline of knowledge, these are great practices for brainstorming ideas, building a product, testing use cases, and validating POCs. You can also test cross-functional delivery. Hackathons often help teams build a POC that they can then move into production after the event.
- Proof of concepts: As previously mentioned, many great AI agents start here. You can iterate through ideas and go through the product development process. But you should always test with customers before moving on to production (more on that in the next section).
- Hiring and mentorship: Depending on your use cases, you might need additional skills. Bring on a mentor to coach existing teams with business and product context on new domains. Mentors or consultants can help with projects on a temporary or ad-hoc basis.
You don’t have to reinvent product management—companies have been launching products for over 50 years—but every role is important to your AI agent project. If you’re missing important skills or roles, you run the risk your AI agent might have the same blind spots. Speaking of risks…
Getting out of POC purgatory: Navigating risk and security
There are a plethora of reasons why your AI agent could get stuck in POC purgatory. In fact, here’s a handy list of challenges you may face. Thanks, Denys, I guess.
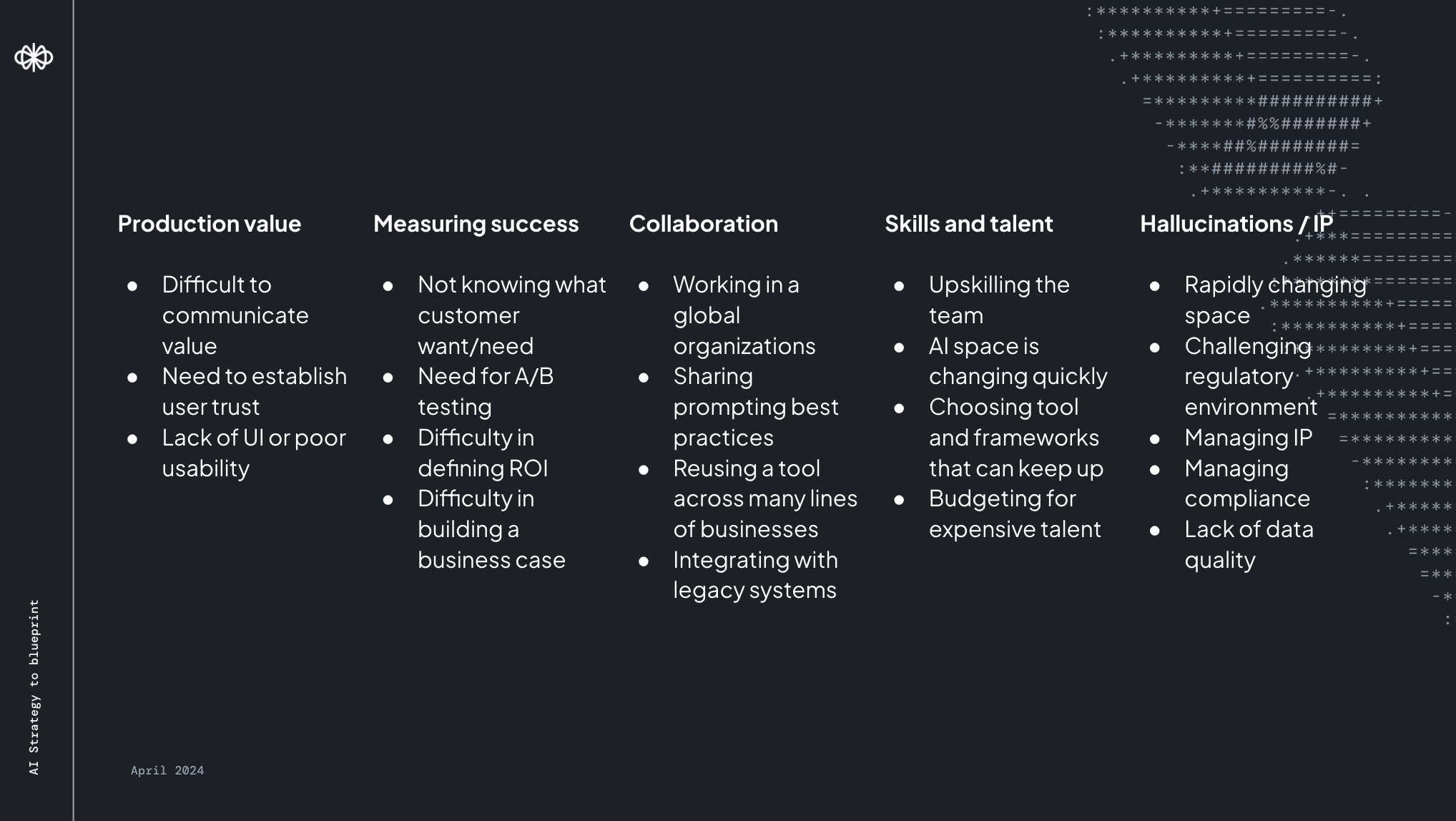
“Now, I don’t share this list to discourage you,” Denys says, “This is what you’ll face as you navigate the risks of building AI agents and treating them like core products.”
Let's address a few of them, so you can too when they come up in your production cycle.
Here’s a common situation. Your CEO says it’s time to build with AI—it’s now your organization’s top priority. But when you dig into how you’re supposed to implement it, your security team says you can’t use AI tools in customer-facing use cases. This may seem like an impassable obstacle, but let's go deeper. You might follow up with security with questions like:
- What are your concerns? Model training with proprietary data?
- Are there personal identifying information or intellectual property concerns?
- Can we use AI tools internally?
- Can we use an LLM to assist with classification tasks?
- If we keep a human in the loop of the process, could we make exceptions?
Once you investigate more, you realize that you can address some of these issues with straightforward solutions. If your security team is concerned about the API using your organization’s data to train the LLM model, bring in legal to review the contract. If security responds that a legal review isn’t enough, then you could suggest that the AI agent only access publicly available data, like data from your website. Other concerns may include compliance with SOC II or GDPR. Your solution could be to offer a clear business case with a POC that reflects lower-risk projects.
Making that leap from POC purgatory to production is about de-risking your AI agent, and there are many ways to do that and still achieve your intended goals. Mapping out your production process (and the security steps you’re taking) will go a long way in gaining buy-in from your legal and security teams. For example, your production calendar could include checkpoints like:
- Review environment (UAT—User acceptance testing): Rollout stakeholder and technical reviews.
- Production internal feature flag: Release to a small set of users to test features before rolling out.
- Private beta review: Validate with the community, select customers, power users and select enterprises. Ensure it’s solving problems and helping those who will be using it.
- General release rollout: Add to core workflow for select general users before full rollout.
- Measurement, testing, iteration: Each time you add a new feature or make a change, you repeat this process. Measure time to production as well, to ensure this process is efficient.
If you take small leaps to get to production—in addition to understanding risks—when you return to your CEO with an update on your AI mandate, your answer will include a production plan with the support of your CIO, CPO, and security team.
Mitigating risk allows you to expand use cases, build better workflows, revisit past decisions, or explore new models with the ability to adapt as things change in the AI space.
Measuring your AI agent’s move and maintain metrics
Most agents fall short because they aren’t being monitored and continually updated to reflect the needs of users. Luckily, Denys has created a handy resource for this—but generally, measuring the success of your agent usually falls under two categories:
1. Move
Metrics your AI agent will directly affect. These metrics will also help inform your iteration process. For example, If you’re measuring:
- Time to first response: You’re quickly acknowledging user issues that come up. If not, you may need to review your AI model and token usage. Focus on not making the workflow too complicated to avoid dropping user messages.
- Resolution rate/speed: You want a faster resolution for customers. That means future iterations you make will prioritize efficiency.
- Ticket throughput: You may realize that your tickets are increasing and your company needs to scale support to keep up, either human or AI.
- Repeat questions: If you have an influx of repeat questions, you may need to focus on debugging your AI agent.
2. Maintain
Metrics that your AI agent should have no impact upon. For example:
- You’d like to use AI to augment your support team so you can maintain a flat team structure and size.
- You don’t want your AI agent to impact customer satisfaction or net promoter score (NPS).
Keeping a close eye on your move and maintain metrics helps guide the iteration conversation and, if your agent is working, proves the ROI of your AI agent investment. But what happens when your agent is not working as planned?
[Don’t know how to measure the success of your AI agent? We can help with that]
Let’s talk tokens—LLMs and the cost of AI agents
First of all, Denys explains, it’s really easy to blame the shiny new AI agent when something goes awry. “Before everyone gets confused about metrics dropping, start with the cause. CSAT may have decreased because of a product change that coincides with the AI agent launch,” he says. Start with the cause of the problem and get more context, it might reveal the issue isn’t the agent, or if it is, the solution can be iterated and solved quickly.
Let’s imagine another common scenario. You’d like to understand the cost per interaction of your LLM—you’re noticing the costs are higher than expected. Firstly, Denys warns against getting comfortable designing an AI agent that calls on your LLM too often. “There's a reason they're called large language models. They’re resource-intensive. If you stack a dollar per interaction and your agent is designed to make 30 LLM calls, you have an AI agent that costs $30 to do a task.” Needless to say, if your finance team hears about it, they’re unlikely to be pleased.
Secondly, you should weigh the engineering costs of optimizing for LLM prompts. If the LLM Haiku costs $0.05 per call, but an engineer is working 10 hours or more on optimizing your AI agent, the cost of their time may not be worth the 900,000 Haiku calls you’d be able to make. “It may seem counterintuitive,” Deny says, “But depending on the model, it may be worth a first production launch with satisfactory accuracy and guardrails, without the hours of labor to optimize for the LLM.”
The good news is that LLM costs are dropping all the time. Today, cheaper models often replace expensive ones in their abilities and can be easily swapped out. Denys advises you to weigh the costs thoughtfully—AI and human—when building and iterating your AI agent. Just like you would any other core product.
[Want to see what AI agent success looks like? Meet Trilogy, the team that automated 60% of their support tickets within 19 weeks.]
AI agents are core products and they should be treated that way
To go from ideas to useful AI agents takes effort and determination. You need to prioritize your use cases and align them closely with what customers want. You need to define success and always be thinking about ROI. And you need to align your AI team with the right skills while helping them collaborate across the organization by mitigating and addressing risk.
But Denys is right, none of this is rocket science. We’re just applying commonly held product management principles to the AI space. The difference now is that we have the chance to make really cool, useful agents.